Blind Deconvolution
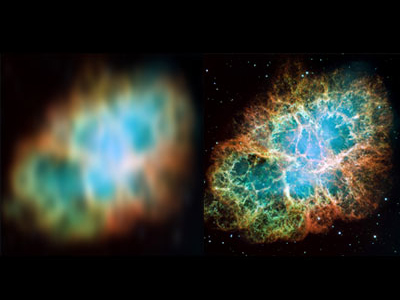
In many imaging applications an observed image can be thought of as a degraded replica of the original one. This degradation is usually nonlinear, thus taking into account phenomena such as quantization, and spatially and temporally varying as in non-uniform motion or imperfect optics. However, for the sake of mathematical tractability the observation model is linearized, thus assuming that the observed image is obtained as the output of a linear, shift invariant system, namely the Point Spread Function (PSF), fed by the original image and contaminated by additive noise. The term “deconvolution” refers to the process of reconstructing the original image from either one or multiple degraded observations, using the information about both the imaging system and the original image. Unfortunately, there are applications where the PSF is either unknown or partially known, and little information is available about the original image. In these cases the original image estimation process is called blind image deconvolution. The field of image deconvolution has several applications in different areas such as image restoration, microscopy, medical imaging, biological imaging, remote sensing, astronomy, nondestructive testing, geophysical prospecting, and many others. There are several factors which motivate the use of blind deconvolution in image processing. In fact, in many practical scenarios it may be expensive, dangerous, or physically impossible either to obtain a priori information about the image to be acquired or information about the PSF. For example, in applications such as astronomy and satellite imaging, the atmospheric turbulence, which partially determines the PSF, is difficult to characterize also because of its time-varying nature. In image photography the PSF of the imaging system (e.g. the lens) is only approximately known. Also in medical imaging applications the PSF of the instruments has to be measured and therefore it is subject to errors. In all these situations as well as in many others, blind deconvolution can be used to generate images of improved quality, which makes this field of research a stimulating and a challenging one.